In recent years, artificial intelligence (AI) has taken significant strides, particularly in the field of natural language processing (NLP). Among the most notable achievements in NLP are large language models (LLMs), which are capable of understanding and generating human-like text. These models have been integrated into various applications, such as chatbots, translation tools, and content creation software, transforming the way we interact with technology. In this article, we will explore the architecture of large language models, how they work, and their impact on industries in India and across the globe.
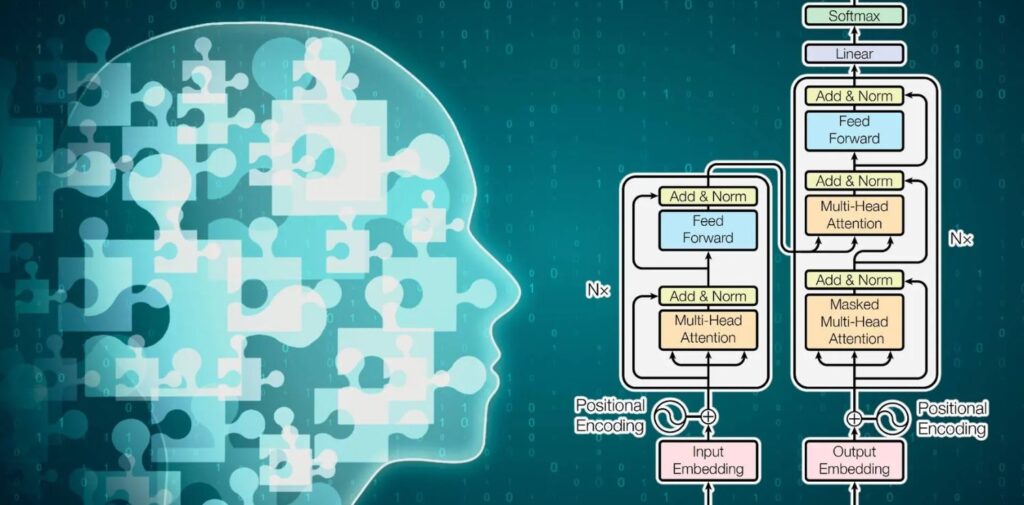
What are Large Language Models?
Large language models (LLMs) are AI models trained on vast amounts of text data to understand and generate human language. They learn patterns in text by processing millions (or even billions) of words from diverse sources such as books, websites, social media, and more. The key feature of LLMs is their ability to predict and generate coherent and contextually relevant text based on the input they receive.
For example, when you ask a language model like OpenAI’s GPT-3 a question, it doesn’t just match keywords; instead, it generates a response based on the patterns and context it has learned during training. The larger the model and the more data it’s trained on, the better it is at understanding complex language tasks, including answering questions, summarizing content, and translating text.
How Do Large Language Models Work?
At the heart of LLMs lies deep learning, a subset of machine learning that uses neural networks to simulate how the human brain processes information. LLMs are built on architectures called transformers, which have revolutionized NLP tasks over the last decade.
Transformers are designed to handle sequences of data (like sentences) and understand the relationships between words in those sequences, even if they are far apart. Traditional models would struggle with long-range dependencies in text, but transformers overcome this limitation by using a mechanism called “self-attention.”
Self-attention allows the model to weigh the importance of each word in a sentence and consider the context of other words, regardless of their position. This is what enables LLMs to generate coherent text and answer questions with a high level of accuracy.
The architecture of LLMs typically includes an encoder-decoder setup. The encoder processes the input text (such as a question), while the decoder generates the output (the answer). However, models like GPT-3 are based on a more streamlined version of the transformer architecture called the decoder-only model, which focuses solely on generating text.
The Role of Training Data in LLMs
Training data plays a crucial role in shaping the performance of large language models. These models are trained on diverse and vast datasets, often spanning multiple languages, topics, and writing styles. In the case of models like GPT-3, the training data includes text from books, articles, websites, and even code repositories.
The diversity of training data allows LLMs to perform well across a wide range of tasks, from answering general knowledge questions to generating creative content. However, the quality of the data is just as important as its quantity. If the model is trained on biased or low-quality data, it may generate biased or inaccurate outputs.
For instance, if an LLM is trained on a dataset that contains stereotypes or harmful language, it may unintentionally reproduce these biases in its responses. This is a major challenge in the development of AI models, and researchers are continuously working to improve the quality and fairness of training data.
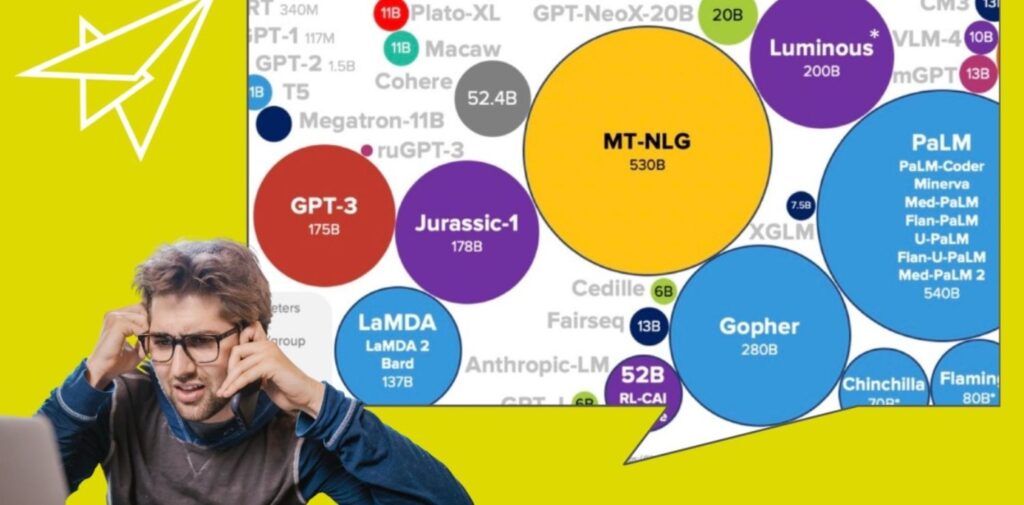
Understanding the Size of Large Language Models
The “large” in large language models refers to the number of parameters they have. Parameters are the weights and connections in a neural network that help the model learn and make predictions. The more parameters a model has, the more complex patterns it can recognize and understand.
For example, GPT-3, one of the largest language models developed by OpenAI, has 175 billion parameters. To put this into perspective, GPT-3 is far larger than its predecessor, GPT-2, which had only 1.5 billion parameters. The size of these models allows them to capture more nuanced aspects of language and perform a wider range of tasks with greater accuracy.
While larger models tend to perform better, they also require significant computational resources to train and deploy. Training a model like GPT-3 can take weeks or even months on powerful hardware, and the cost of training such models can run into millions of dollars. This is why only a few companies and research institutions with substantial resources can afford to develop and maintain such models.
The Impact of Large Language Models in India and the World
The development of large language models has profound implications across various sectors, and their impact is being felt worldwide, including in India. These models are transforming industries such as customer service, content creation, education, healthcare, and more.
In India, where there is a wide diversity of languages, LLMs have the potential to bridge language barriers and improve communication across different regions. For instance, language models can be used to create more accurate translation tools, helping people communicate in different Indian languages such as Hindi, Tamil, Telugu, and others. This is particularly useful in a country with over 22 official languages and a population that speaks hundreds of dialects.
Moreover, businesses in India are increasingly adopting AI-powered tools, such as chatbots and virtual assistants, which are powered by LLMs. These tools can handle customer queries in a variety of languages and provide personalized recommendations, improving customer experience and reducing operational costs.
In education, LLMs can assist in creating intelligent tutoring systems that offer personalized learning experiences for students. These systems can answer students’ questions, explain complex concepts, and even help with homework, making education more accessible and efficient.
In healthcare, LLMs are being used to process medical records, assist in diagnostics, and provide support for healthcare professionals. By analyzing vast amounts of medical data, LLMs can help doctors make better-informed decisions and improve patient care.
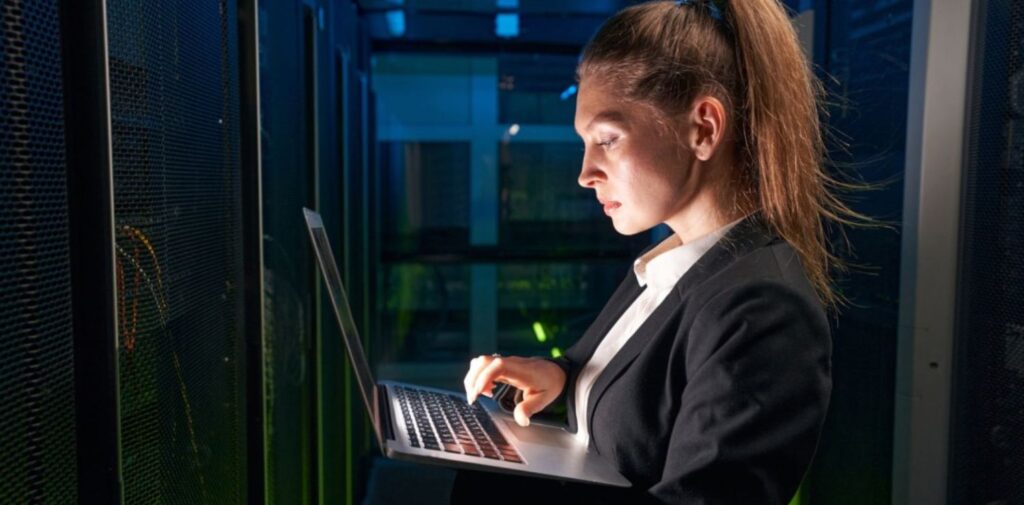
Challenges and Ethical Considerations of Large Language Models
Despite their impressive capabilities, large language models come with several challenges and ethical concerns. One of the biggest challenges is their energy consumption. Training and running LLMs require enormous computational power, which has a significant environmental impact. Researchers are working on developing more energy-efficient models, but this remains a pressing issue.
Another challenge is the potential for misuse. LLMs are capable of generating realistic-sounding text, which can be used to create fake news, misinformation, and malicious content. As LLMs become more advanced, ensuring that they are used responsibly and ethically becomes increasingly important. Developers and policymakers must work together to establish guidelines and regulations for the ethical use of these technologies.
Bias is another major concern. Because LLMs learn from data, they can unintentionally perpetuate biases present in the data they are trained on. These biases can manifest in the form of gender, racial, or cultural biases, which can lead to harmful outcomes if not addressed properly.
Conclusion
Large language models represent a remarkable advancement in AI and natural language processing. Their ability to understand and generate human-like text has opened up new possibilities for automation, communication, and problem-solving across various industries. While these models offer immense potential, they also come with challenges related to energy consumption, bias, and ethical considerations. As the technology continues to evolve, it is crucial that researchers, developers, and policymakers work together to ensure that LLMs are used responsibly and for the benefit of society.
In India, where language diversity and technological adoption are growing rapidly, large language models have the potential to transform various sectors, from education to healthcare. By understanding their architecture and implications, we can better appreciate the immense possibilities they bring to the table and work towards harnessing their power in a responsible and sustainable manner.