Artificial Intelligence, or AI, is becoming a part of our daily lives in ways we often don’t notice. From getting suggestions on what to watch next on Netflix to using voice assistants like Alexa or Google Assistant, AI is everywhere. In India, we are seeing AI being used in government projects, agriculture, banking, healthcare, and even in schools. But one big question still remains — Can we trust AI?
For AI to be truly helpful and accepted by society, it must be explainable. People should be able to understand why an AI system took a certain decision, especially in sensitive areas like health, law, and finance. This is where Explainable AI (XAI) comes into play. Let’s understand this concept and see how we can aabuild trust in AI through explainability.
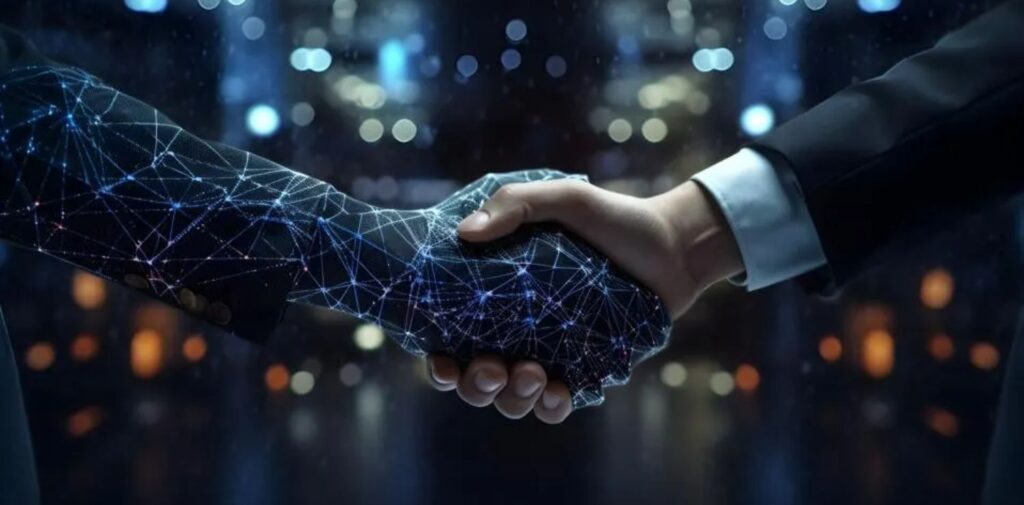
Why Trust Matters in AI
Trust is the foundation of any technology that impacts human lives. Just like we trust doctors to make the right diagnosis or banks to keep our money safe, we need to trust AI systems to make fair and accurate decisions. But unlike a doctor or a bank manager, an AI system is often like a black box. It gives an output, but we don’t always know how or why it arrived at that decision.
For example, if an AI system rejects a loan application, the applicant has every right to know the reason. If the system cannot explain why it rejected the loan, how can the applicant trust it? In a country like India, where financial inclusion is a big goal, such lack of transparency can lead to serious problems.
What is Explainable AI (XAI)?
Explainable AI means building AI systems in such a way that their decisions can be easily understood by humans. This doesn’t mean that everyone has to become a data scientist. It simply means that if a decision affects someone, they should be able to understand the reason behind it in simple terms.
There are two main types of explainability in AI:
- Global Explainability: This explains how the whole AI model works. For example, it tells you which factors are important for the model in general.
- Local Explainability: This explains why the model made a specific decision for a particular case. For example, why a student didn’t qualify for a scholarship according to an AI tool.
In India, where we have such a large and diverse population with different languages and education levels, local explainability becomes even more important. A farmer using an AI app to predict crop yields or a student checking exam results through an AI-based system should be able to understand the logic behind the results.
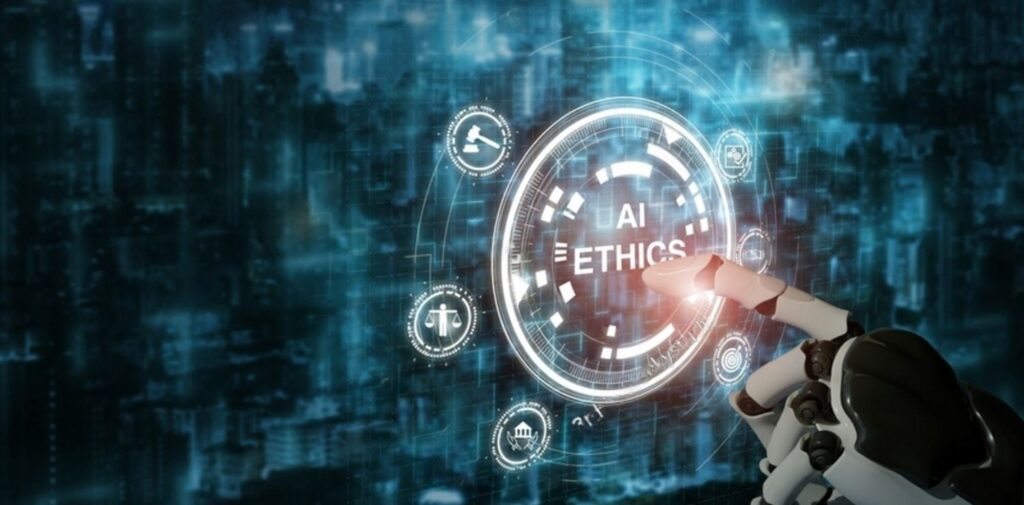
Real-Life Applications and Need for Explainability in India
AI is slowly becoming a part of many important sectors in India. Let’s take a look at a few examples where explainability becomes very important:
- Healthcare: AI tools are being used to diagnose diseases, predict health risks, and even assist in surgeries. If a system predicts that someone is at high risk of cancer, the patient needs to know why. A clear explanation builds trust and also helps doctors in taking better decisions.
- Banking and Finance: Many banks in India are using AI to check credit scores, detect fraud, and approve loans. But if someone is denied a loan or flagged for fraud, they must be able to understand the reason. If the system is biased or faulty, people’s lives can be seriously affected.
- Education: AI is being used for evaluating exams, personalized learning, and even career counseling. But a student must know why the AI recommended a certain career or gave low marks. Otherwise, students and parents may not trust these systems.
- Government Services: AI is being used in schemes like Digital India, Swachh Bharat, and smart city projects. For public trust, citizens should understand how AI is making decisions in these areas.
Challenges in Making AI Explainable
Making AI systems explainable is not an easy task. There are many challenges in the Indian context:
- Complex Models: Many modern AI models are very complex. Deep learning systems, for example, use layers and layers of algorithms which are difficult to explain in simple terms.
- Lack of Awareness: Many users in India, especially in rural areas, may not know how AI works. Even if an explanation is given, it has to be in very simple language and in local dialects.
- Bias and Fairness: Sometimes, AI systems can develop biases based on the data they are trained on. In a diverse country like India, data may be biased due to regional, economic, or gender factors. These biases must be explained and corrected.
- Lack of Regulations: India is still in the early stages of developing laws and policies around AI. Without clear rules, companies may not feel responsible for making their systems explainable.
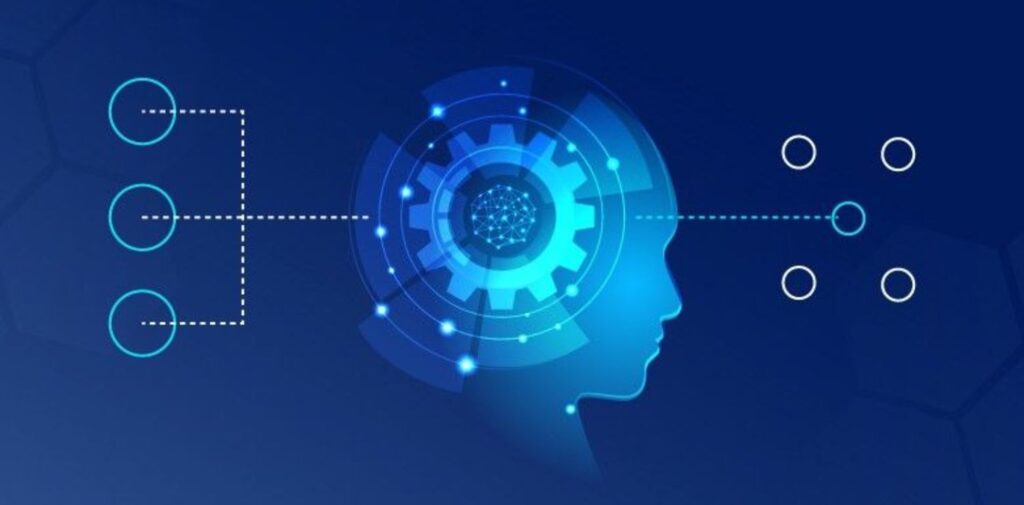
Steps to Build Explainable AI Systems in India
To make AI systems more trustworthy and explainable, we need to take certain steps, especially keeping the Indian population in mind:
- Simple and Clear User Interfaces: The results of AI systems should be presented in a simple manner. Use of regional languages, icons, and visual explanations can help a lot.
- Human-in-the-Loop Approach: This means including humans in the decision-making process. For example, an AI can give a recommendation, but a human can make the final decision.
- Ethical AI Guidelines: India should promote ethical AI usage through national policies. Companies and developers should follow guidelines to make AI fair, transparent, and explainable.
- Education and Awareness: Basic AI education should be included in schools and colleges. Citizens should know how AI works and how it can affect their lives.
- Open-Source and Public AI Tools: Government can develop and promote open AI tools that are explainable and easy to use. This will help smaller businesses and startups as well.
Conclusion: The Future of Trust in AI in India
As AI becomes more powerful and widely used, the question of trust becomes even more important. In a country as large and diverse as India, AI has the power to transform lives — but only if people can trust it. Explainable AI is the key to that trust.
By building AI systems that are easy to understand, fair, and transparent, we can ensure that everyone — whether a student in a village, a doctor in a hospital, or a farmer in a field — can rely on AI with confidence. The journey to trust starts with clear explanations. And in a country like ours, that’s not just a technical need — it’s a social responsibility.
If we focus on making AI more human-friendly and understandable, we can truly use it as a tool for development, growth, and inclusion across all parts of India.