In today’s digital world, artificial intelligence (AI) has become an important part of our lives. From voice assistants like Alexa and Siri to online shopping recommendations, AI is everywhere. But have you ever wondered how AI becomes so smart? The secret behind its intelligence is data. Data is like food for AI. The more data it gets, the better it performs. However, there’s an ongoing debate in the world of AI — what is more important, the quantity of data or the quality of data? In this article, we will understand the role of data in AI and why both quality and quantity matter, especially from an Indian perspective.
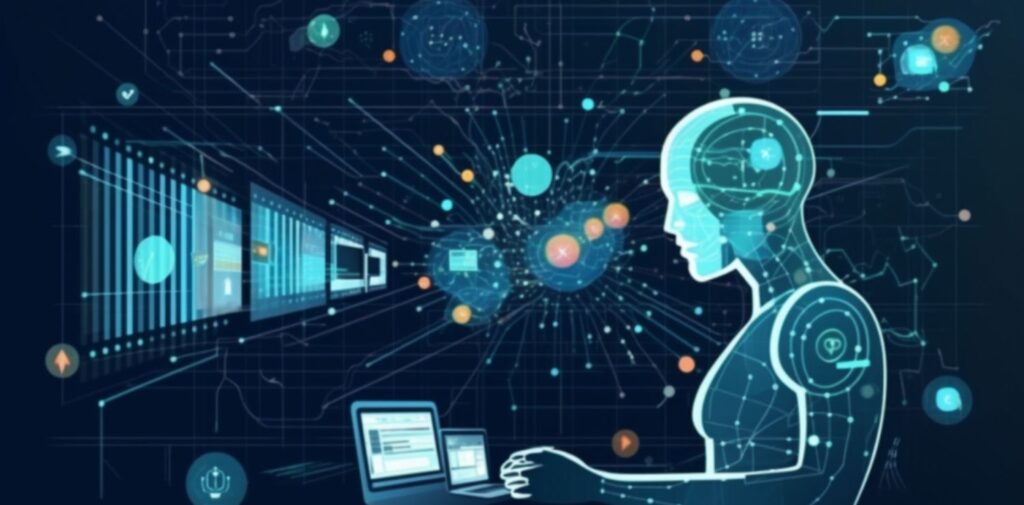
Understanding how data powers AI
AI works by learning from examples. These examples come from data. Let’s take a simple example of teaching an AI system to identify fruits. You show the system many images of apples, bananas, and oranges. The system learns the shape, color, and texture of each fruit by analyzing the data. The more data you give, the better it understands. But if your data is incorrect or unclear — like an image of an apple labeled as an orange — then the AI will get confused.
This is why data is so important. AI is like a student, and data is the study material. Good study material helps the student score well, but wrong material can lead to failure. So, we need to ensure that the data we feed into AI is accurate and useful.
Quantity of data – More is better?
One side of the argument says that the more data you have, the better your AI system will become. This is because large amounts of data help AI learn more patterns and variations. For example, if you are building a language translation app for Hindi and English, having thousands of sentences in both languages helps the AI understand grammar, sentence structure, and word meanings better.
In India, where we have many regional languages and dialects, collecting large amounts of data is helpful. More data from different parts of the country can make AI systems more inclusive and accurate for everyone. A chatbot trained only on Delhi Hindi may not understand the Bhojpuri or Marwari version of Hindi. So, more data from different regions ensures that AI works well for all Indians.
But there is a catch. If you just keep feeding more and more data without checking its correctness, the AI will learn wrong things. This is where the concept of quality comes in.
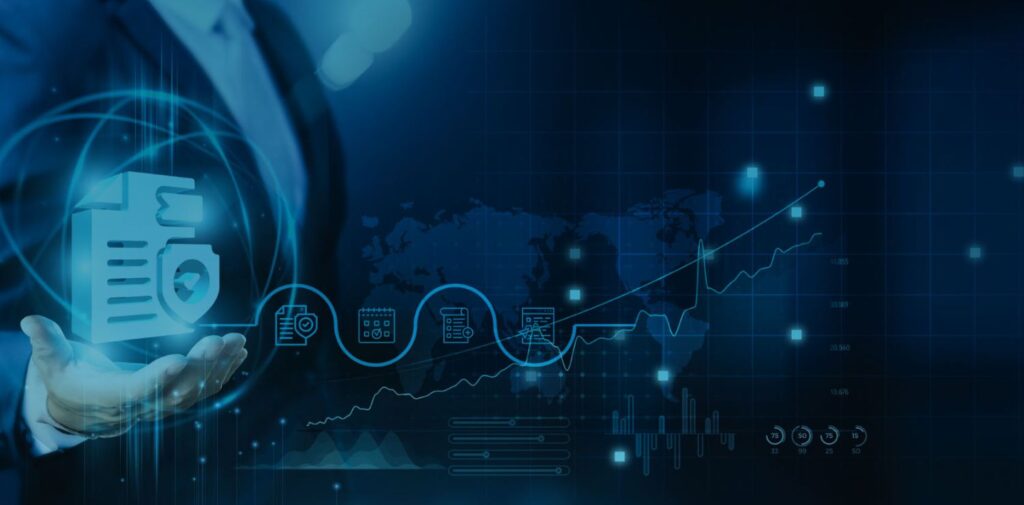
Quality of data – Clean and correct data matters
Let’s imagine you are preparing for an exam. You can either read one excellent textbook or ten badly written books full of mistakes. Which one would you choose? Obviously, the good one. The same logic applies to AI. Giving it correct and clean data is better than giving it tons of wrong or noisy data.
For example, in the healthcare sector in India, if you are building an AI tool to detect diseases from X-ray images, the images must be clear, properly labeled, and reviewed by doctors. If the data is poor quality, the AI might make dangerous mistakes. This can have serious consequences in fields like medicine, banking, and law.
So, even if we have a smaller amount of data, if it is accurate and well-structured, the AI can still perform very well. In many cases, quality is more important than quantity.
Balancing quality and quantity – The ideal solution
Now that we understand the importance of both quality and quantity, the real solution is to find the right balance. Imagine you are building an AI model to recognize Indian food from pictures. You need a large variety of images — dosa, biryani, samosa, idli, etc. This covers the quantity. But you also need the images to be labeled properly and taken from different angles and lighting conditions. This covers the quality.
Indian companies and startups are working hard to build large datasets that are also high in quality. Government initiatives like Digital India and private projects like AI for Bharat are helping in this mission. The goal is to create AI that works for every Indian, whether they live in Mumbai or a small village in Assam.
Challenges in India related to AI data
India faces some unique challenges when it comes to collecting data for AI. First, there is a lack of properly labeled data in regional languages. Most AI tools are trained on English data, which limits their use in rural areas. Second, there is a shortage of skilled people who can clean and organize data. Third, privacy is a big issue. People are becoming more aware of how their data is being used, and companies need to handle it responsibly.
To overcome these issues, we need a strong system for data collection, labeling, and privacy protection. Training programs can help more people learn about data handling, and strict data protection laws can keep people’s personal information safe.
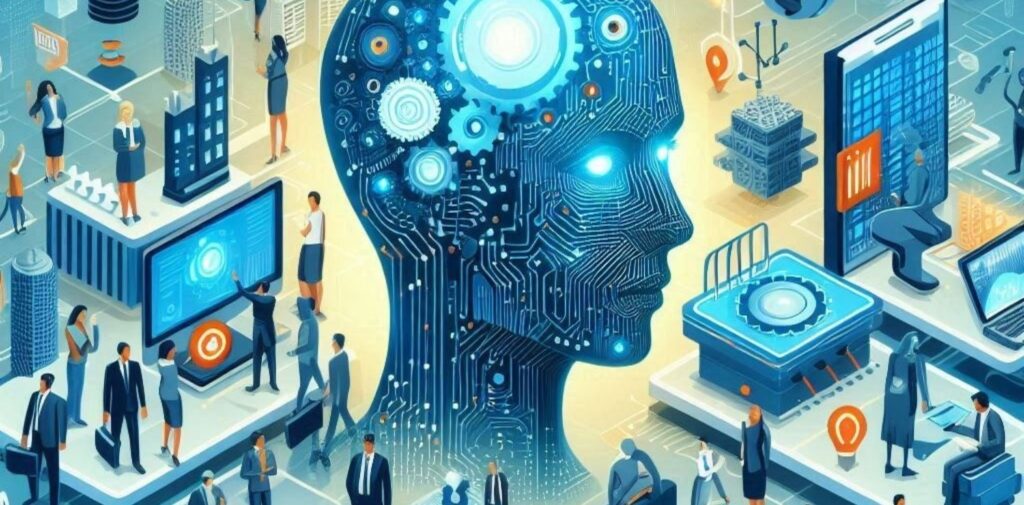
The future of AI in India depends on better data
India has a huge opportunity to become a global leader in AI. We have a large population, many languages, and diverse needs — all of which can be supported with the right kind of AI tools. But to build such tools, we need to focus on improving our data systems.
Government policies should encourage the creation of open and shared datasets. Educational institutions can teach students how to manage data. Startups can use smart methods to collect clean and useful data. Together, these efforts will make sure that AI in India becomes not only powerful but also trustworthy and fair.
Conclusion:
In conclusion, both quality and quantity of data are important for building good AI systems. If you focus only on quantity, you may get confused results. If you focus only on quality with too little data, your system might be weak. The smart way is to balance both. For India, this balance will help create AI that truly understands and supports its people in every language, region, and sector.
AI is the future, and data is its fuel. Let’s make sure we are giving it the right fuel to drive India ahead.