Artificial Intelligence (AI) has become an essential part of our daily lives. From voice assistants like Alexa and Siri to recommendation systems on platforms like YouTube and Netflix, AI is all around us. But have you ever wondered how these systems learn to perform tasks, make decisions, and predict outcomes? The answer lies in datasets. In simple terms, a dataset is a collection of data that is used to teach AI models how to understand and solve problems. Just as humans learn from experience, AI learns from datasets.
In this article, we will explore the importance of datasets in training AI models, why they are crucial, and how they impact the performance and accuracy of AI systems. Whether you’re a student, a professional, or someone interested in AI, this article will give you valuable insights into this fundamental aspect of AI technology.
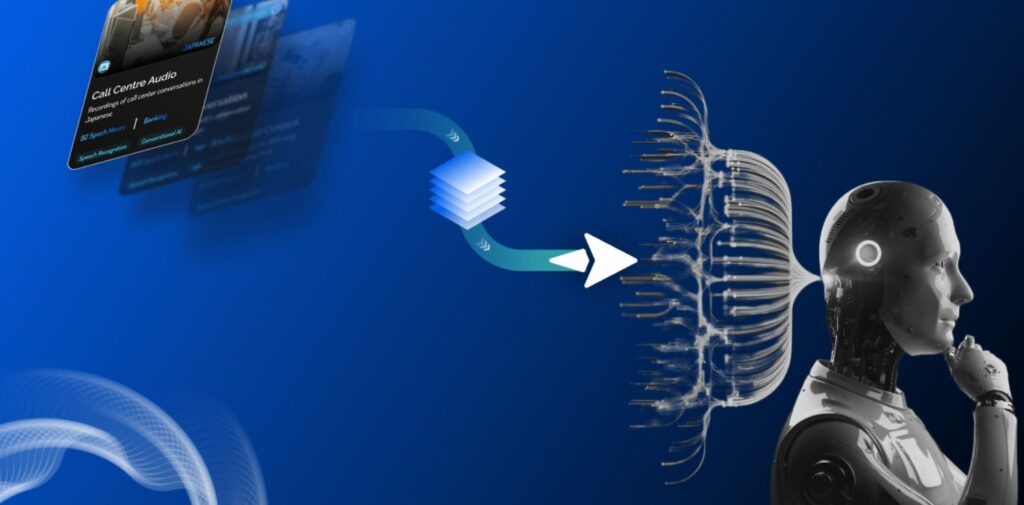
What are Datasets in AI?
A dataset is a large collection of data that is used to train machine learning or AI models. This data can come in various forms such as numbers, text, images, or audio. For example, an AI model that detects whether a photo contains a cat or not will require a dataset of labeled images (i.e., images that are clearly marked as containing a cat or not). The AI model will learn from these examples and improve its accuracy over time.
The role of datasets is very similar to that of a teacher guiding a student. Without the right examples and the right amount of data, the AI model cannot learn effectively. The better and more diverse the dataset, the more accurate and reliable the AI system will be.
How Datasets Help Train AI Models
AI models, specifically machine learning algorithms, work by learning patterns and relationships from the data they are provided. When an AI model is fed a dataset, it starts identifying patterns in the data and uses these patterns to make decisions. These models typically undergo two stages: training and testing.
- Training Phase: During this phase, the AI model learns from a large dataset. For example, if the AI is being trained to identify pictures of dogs, it will be shown thousands of pictures of dogs along with the label “dog.” Over time, the model learns the common features of a dog—its shape, size, fur, and other characteristics. The more data the AI gets, the better it can refine its understanding.
- Testing Phase: After training, the model is tested with a new dataset that it has never seen before. This helps assess how well the model can apply its learned knowledge to new, unseen data. If the AI is able to identify dogs in the testing data accurately, it means it has learned well from the training dataset.
The Significance of Quality and Quantity in Datasets
For AI to function well, the quality and quantity of data used for training are very important. Let’s take a look at both of these aspects:
- Quality of Data: The data provided to the AI model must be accurate and relevant. For instance, if you’re training a model to predict weather patterns, the data should include accurate records of temperature, humidity, wind speed, and other weather-related factors. If the data is incomplete, outdated, or incorrect, the AI model will not learn effectively and may produce incorrect results.
- Quantity of Data: The amount of data available is also crucial. Generally speaking, the more data you have, the better the AI model will perform. This is because having a larger dataset allows the AI model to recognize more patterns and relationships. If you have just a few images of dogs, the AI might not learn to identify all types of dogs. However, if you have thousands of images from various environments, angles, and lighting conditions, the AI will become much more accurate.
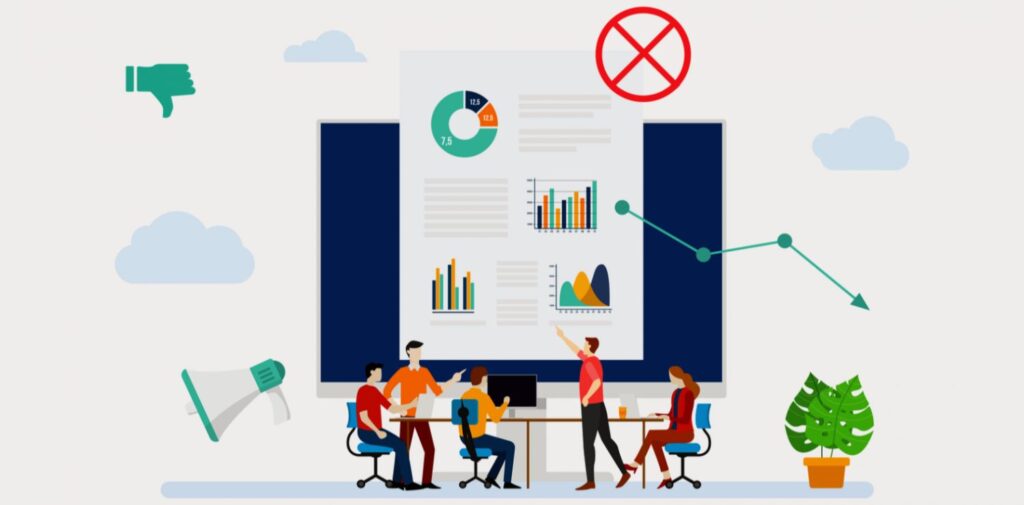
Diverse Datasets Lead to Better Performance
A key to building a successful AI system is ensuring that the dataset used for training is diverse. Diverse datasets contain a wide range of examples that can help the AI model learn from various scenarios. For instance, if you’re training an AI system to recognize faces, it’s important that the dataset includes images of people from different ethnicities, ages, and genders. This ensures that the model doesn’t develop biases or fail to recognize faces that are different from the ones it has seen during training.
In India, a country known for its diversity, AI systems must be trained with datasets that represent people from various cultural, linguistic, and social backgrounds. For example, speech recognition models need to be trained on datasets that include different Indian accents, languages, and dialects to ensure they work accurately for everyone. Without diverse datasets, AI systems might perform poorly or even discriminate against certain groups of people.
Challenges in Creating Good Datasets
While datasets are crucial for training AI models, creating high-quality datasets can be a challenging task. Here are some common challenges faced when creating datasets for AI:
- Data Collection: Gathering a large amount of relevant data is often difficult and time-consuming. In many cases, you need to collect data from various sources, clean it, and organize it for use in training. For example, training a model to detect diseases in medical images requires a large collection of labeled medical images from hospitals, which can be difficult to obtain.
- Data Labeling: Labeling the data correctly is another challenge. If you’re training a model to recognize objects in images, each image must be accurately labeled with the object it contains. This can be a tedious process and often requires manual effort. Incorrect labeling can lead to errors in the model’s learning process.
- Bias in Data: Another major challenge is bias in the dataset. If the data used for training is biased or unbalanced, the AI model will learn those biases. For example, if a facial recognition model is trained only on images of light-skinned people, it will not perform well when trying to recognize people with darker skin tones. Bias in datasets can lead to unfair or discriminatory outcomes, which is a major concern in AI development.
- Privacy Concerns: Datasets often contain sensitive information, such as personal data, health records, or financial details. It’s important to ensure that the data used for training AI models is collected and used ethically, with the consent of the individuals involved. In India, data privacy laws such as the Personal Data Protection Bill aim to address these concerns and ensure that personal data is handled responsibly.
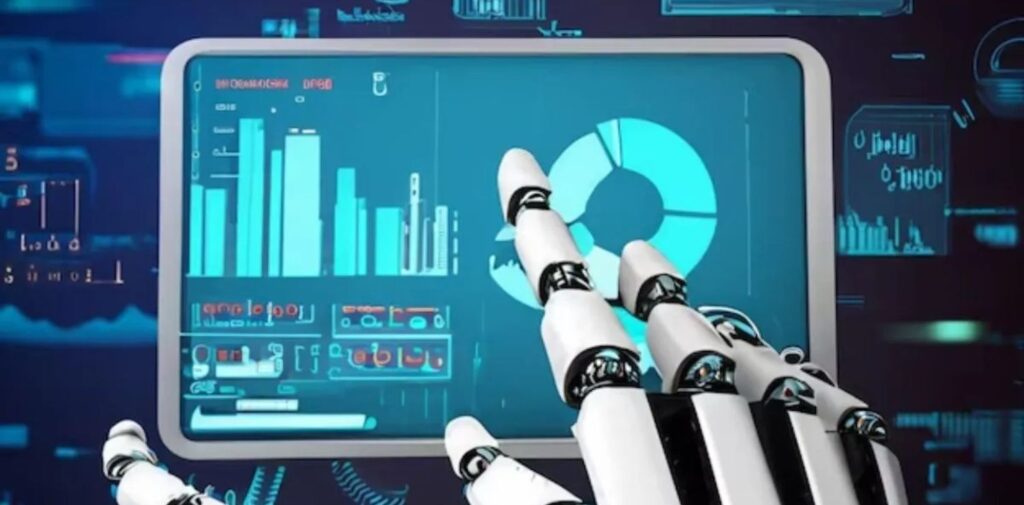
How AI Datasets are Revolutionizing Industries in India
In India, AI and machine learning are making waves across various sectors, and datasets play a key role in this transformation. Here are some examples of how AI models, trained on quality datasets, are benefiting industries in India:
- Healthcare: AI models trained on large datasets of medical images and patient records are helping doctors diagnose diseases like cancer, diabetes, and tuberculosis more accurately. These models can analyze medical data faster and more efficiently than humans, enabling early detection and better treatment outcomes.
- Agriculture: AI models are being used to predict crop yields, identify pests, and optimize farming practices. Datasets containing information about weather patterns, soil quality, and crop growth are helping farmers make data-driven decisions to improve productivity.
- Finance: In the financial sector, AI models trained on datasets of market trends, customer transactions, and economic indicators are being used for fraud detection, credit scoring, and risk assessment. These models are helping banks and financial institutions provide better services and make smarter decisions.
- E-commerce: AI-powered recommendation systems, trained on datasets of customer preferences and behavior, are helping online retailers like Amazon and Flipkart provide personalized shopping experiences. These systems analyze customer data to suggest products that are most likely to be purchased, improving sales and customer satisfaction.
Conclusion: Why Datasets Matter in AI
In conclusion, datasets are the backbone of AI models. They are essential for training AI systems to understand, learn, and make decisions. The quality, quantity, and diversity of datasets directly impact the performance and accuracy of AI models. As AI continues to revolutionize industries in India and around the world, creating better datasets and addressing challenges like bias and data privacy will be key to ensuring that AI systems are fair, reliable, and beneficial for everyone.
As India continues to develop as a hub for AI innovation, the importance of high-quality datasets will only grow. Whether it’s improving healthcare, optimizing agriculture, or enhancing customer experiences, datasets will continue to be the foundation upon which AI models are built.